Sapienza NLP @ NAACL 2024
2 papers at NAACL!
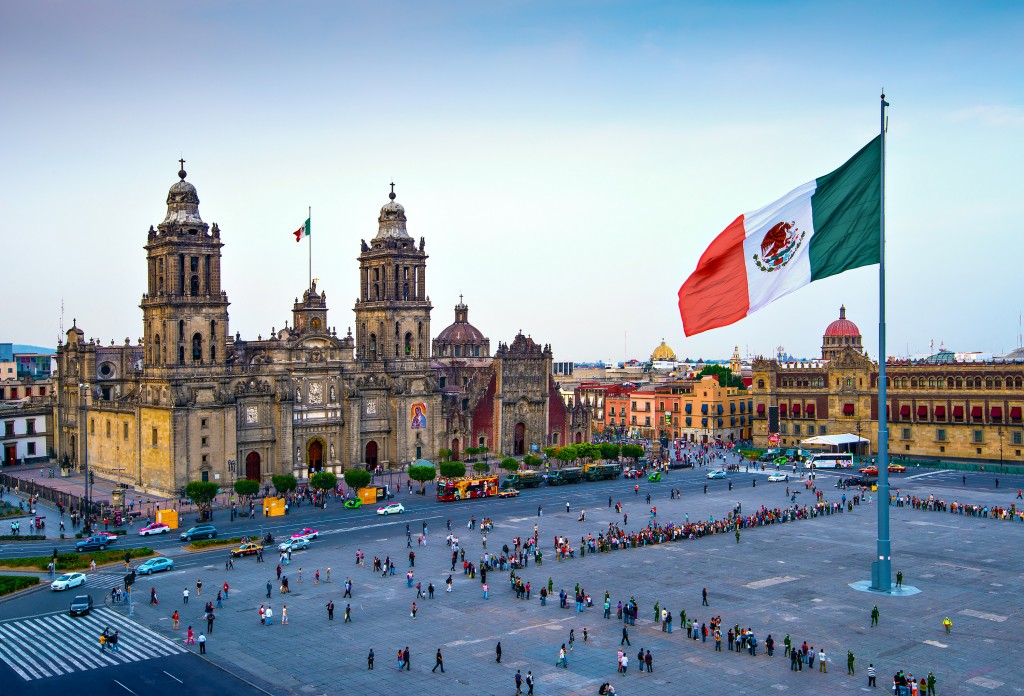
CNER: Concept and Named Entity Recognition
by G. Martinelli, F. Molfese, S. Tedeschi, A. Fernández-Castro, R. Navigli
Named entities – typically expressed via proper nouns – play a key role in Natural Language Processing, as their identification and comprehension are crucial in tasks such as Relation Extraction, Coreference Resolution and Question Answering, among others. Tasks like these also often entail dealing with concepts – typically represented by common nouns – which, however, have not received as much attention. Indeed, the potential of their identification and understanding remains underexplored, as does the benefit of a synergistic formulation with named entities. To fill this gap, we introduce Concept and Named Entity Recognition (CNER), a new unified task that handles concepts and entities mentioned in unstructured texts seamlessly. We put forward a comprehensive set of categories that can be used to model concepts and named entities jointly, and propose new approaches for the creation of CNER datasets. We evaluate the benefits of performing CNER as a unified task extensively, showing that a CNER model gains up to +5.4 and +8 macro F1 points when compared to specialized named entity and concept recognition systems, respectively. Finally, to encourage the development of CNER systems, we release our datasets and models at https://github.com/Babelscape/cner.
MOSAICo: a Multilingual Open-text Semantically Annotated Interlinked Corpus
by S. Conia, E. Barba, A. C. Martinez Lorenzo, P. Huguet Cabot, R. Orlando, L. Procopio, R. Navigli
Several Natural Language Understanding (NLU) tasks focus on linking text to explicit knowledge, including Word Sense Disambiguation, Semantic Role Labeling, Semantic Parsing, and Relation Extraction. In addition to the importance of connecting raw text with explicit knowledge bases, the integration of such carefully curated knowledge into deep learning models has been shown to be beneficial across a diverse range of applications, including Language Modeling and Machine Translation. Nevertheless, the scarcity of semantically-annotated corpora across various tasks and languages limits the potential advantages significantly. To address this issue, we put forward MOSAICo, the first endeavor aimed at equipping the research community with the key ingredients to model explicit semantic knowledge at a large scale, providing hundreds of millions of silver yet high-quality annotations for four NLU tasks across five languages. We describe the creation process of MOSAICo, demonstrate its quality and variety, and analyze the interplay between different types of semantic information. MOSAICo, available at https://github.com/SapienzaNLP/mosaico, aims to drop the requirement of closed, licensed datasets and represents a step towards a level playing field across languages and tasks in NLU.