SapienzaNLP @ COLING 2020
2 papers at COLING 2020!
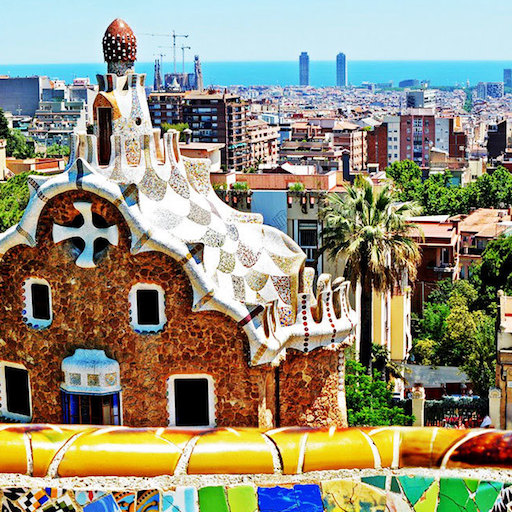
Bridging the Gap in Multilingual Semantic Role Labeling: a Language-Agnostic Approach
by Simone Conia and Roberto Navigli
Recent research indicates that taking advantage of complex syntactic features leads to favorable results in Semantic Role Labeling. Nonetheless, an analysis of the latest state-of-the-art multilingual systems reveals the difficulty of bridging the wide gap in performance between high-resource (e.g., English) and low-resource (e.g., German) settings. To overcome this issue, we propose a fully language-agnostic model that does away with morphological and syntactic features to achieve robustness across languages. Our approach outperforms the state of the art in all the languages of the CoNLL-2009 benchmark dataset, especially whenever a scarce amount of training data is available. Our objective is not to reject approaches that rely on syntax, rather to set a strong and consistent language-independent baseline for future innovations in Semantic Role Labeling.
Conception: Multilingually-Enhanced, Human-Readable Concept Vector Representations
by Simone Conia and Roberto Navigli
To date, the most successful word, word sense, and concept modelling techniques have used large corpora and knowledge resources to produce dense vector representations that capture semantic similarities in a relatively low-dimensional space. Most current approaches, however, suffer from a monolingual bias, with their strength depending on the amount of data available across languages. In this paper we address this issue and propose Conception, a novel technique for building language-independent vector representations of concepts which places multilinguality at its core while retaining explicit relationships between concepts. Our approach results in high-coverage representations that outperform the state of the art in multilingual and cross-lingual Semantic Word Similarity and Word Sense Disambiguation, proving particularly robust on low-resource languages.